Your Options When Choosing a Semantic Data Platform
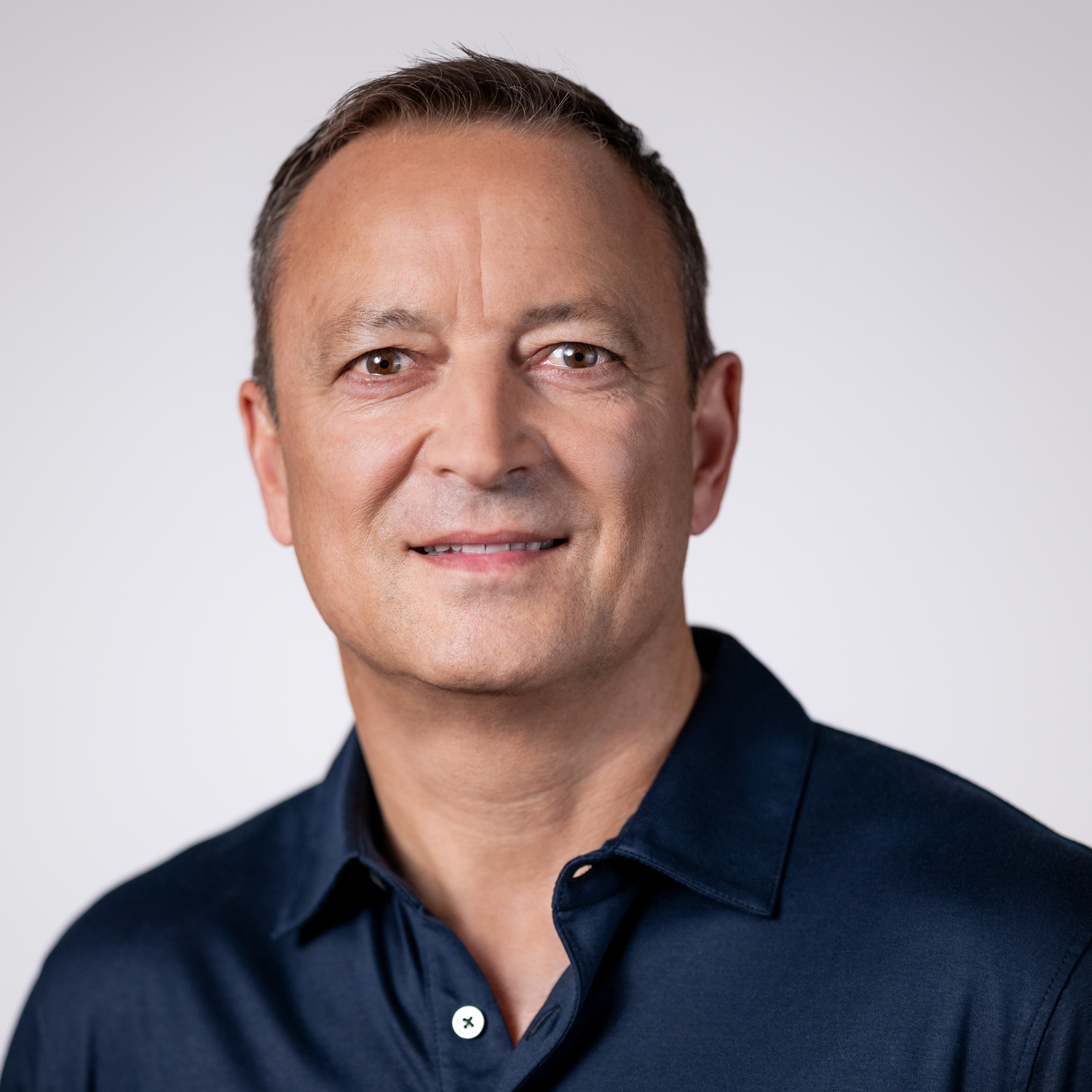
Christian Sauter, CEO
Let’s explore the options available to ambitious companies and public institutions looking to fully leverage the potential of their data. Motivations may range from addressing specific operational challenges to pursuing data-driven business models. In any case, a semantic data platform supporting reuse, insights, and action is the key ingredient for success. We’re talking about an enterprise - grade platform - not merely a graph database, although such databases are a basic component of these platforms. Let’s also examine the advantages and disadvantages of each option.
Our product Bardioc is an extremely scalable platform that encompasses all requirements for professional, ontology-based development and operation of a data platform. Initially, we developed Bardioc to manage data for our problem solving AI, or machine reasoning engine. This software is the result of substantial investment - several hundred person-years in research and development.
At this point, I assume there is a clear understanding why a semantic data platform in needed. Companies that recognize this need understand there is value in their data. Unfortunately so far, the value extraction and use of data have been minimal. Traditional enterprises are often disrupted by startups or tech giants because they lack the ability to leverage and understand their data, let alone enable autonomous actions based on their only true asset - their experience.
The primary function of a semantic data platform is to store and interpret data independently of its original source and to integrate data from various sources. The unique aspect of data, unlike physical products, is that it can be used repeatedly without diminishing its value. In fact using data creates even more data. A semantic data platform is the foundation for reuse, which is essential for extracting any meaningful value from data. By combining data comprehensively from multiple sources, businesses can gain insights into fundamental mechanisms across any business process, market, or client relationship. A semantic data platform is therefore the only way to generate true insight across all levels - from granular detail to the big picture.
The Options
Approaches that focus narrowly on specific use cases, such as in the legal or pharmaceutical sectors, are insufficient for the strategic, overarching needs described above. A semantic data platform that supports data reuse is essential for broader, strategic considerations - something not covered by specialized approaches.
Option 1: Traditional Approach, e.g., Data Lakes
Traditional approaches, like data lakes are widely available, with numerous offerings. Especially cloud-based services such as those from Snowflake, promise manageable costs. However, as we assess these solutions critically, we’ve observed that these approaches often fall short of fulfilling strategic enterprise needs beyond simply storing data for many industry leading organizations. Particularly in terms of data reuse, these solutions present significant limitations, making it challenging to achieve strategic objectives.
You find a comparative analysis of data lakes and semantic graph data platforms here.
Option 2: Custom – Do It Yourself
Ambitious IT departments may attempt to build a custom semantic data platform using open-source software and in-house development. As in many development projects the devil is in the detail and serious problems only arise once you are processing a certain amount of data or try to make a specific calculation. This is also why simply deploying a single-node graph database works for a simple and fairly small dataset, but you will quickly find out that keeping performance for queries, traversals and searches at an acceptable level while the size of your dataset scales linearly and the size of its interconnectedness scales exponentially creates interesting programming problems. After more than two decades of intensive research and development, we urge caution with these DIY approaches.
Option 3: Custom – Via Global System Integrator
This option is similar to the DIY approach, but with the critical difference that a trusted system integrator oversees the development as a contract project. We frequently see these initiatives undertaken by major international system integrators. It’s easy to imagine the scale of budgets and timelines these projects require. Given current project management paradigms these projects are often not driven by the strategic desire to use your data as an asset, but need to produce short term results and end up being a deployment of several independent MVPs which in term do not lend themselves to creating a dataset that represents your world and allows for general reuse within your organization.
Option 4: Palantir
Palantir The US company Palantir is known for its platforms, notably Foundry and Gotham, which are backed by strong relationships with various US institutions as clients and financial supporters. For organizations that prioritize a certain level of sovereignty, Palantir’s offerings may be viewed critically. Undoubtedly, the technology is very powerful, but it also demands substantial financial commitment for both initial implementation and long-term use. Also Palantir‘s primary focus is on creating insight from your data, while automation and efficiency only come in as secondary goals.
Option 5: Bardioc
Our platform, Bardioc, is comparable to Palantir’s offerings when considering the foundational aspects of a semantic data platform. In terms of analytics, Bardioc does not currently offer a portfolio as extensive as Palantir’s because our primary focus has always been action, i.e. automation and efficiency, rather than insights for analysts. We believe Bardioc has unique functionality with our own AI reasoning engine and the ability to integrate other AI technologies. Palantir is marketing its lack of proprietary AI technology as market independence. Bardioc can be deployed on-premises or as a SaaS solution and supports key sovereignty objectives.
Evaluation
What criteria should organizations use when deciding between these options? We believe the following points are particularly relevant:
- Time to Market
- Risk of Implementation (technical risks)
- Data Re-usability
- Sovereignty
- Innovation Potential (AI capabilities)
We have translated these criteria into three matrices, where each of the five options has been evaluated and scored. These matrices reflect our extensive market experience and observations we made at leading European companies. The picture may be different in Asia-Pacific or North America, and we will examine these regions shortly.
For any further questions or to discuss the topic further, please feel free to contact me at the email address christian.sauter@almato.com.