Semantic Recommendation Engines: The Future of Personalized Recommendations in Retail and E-Commerce
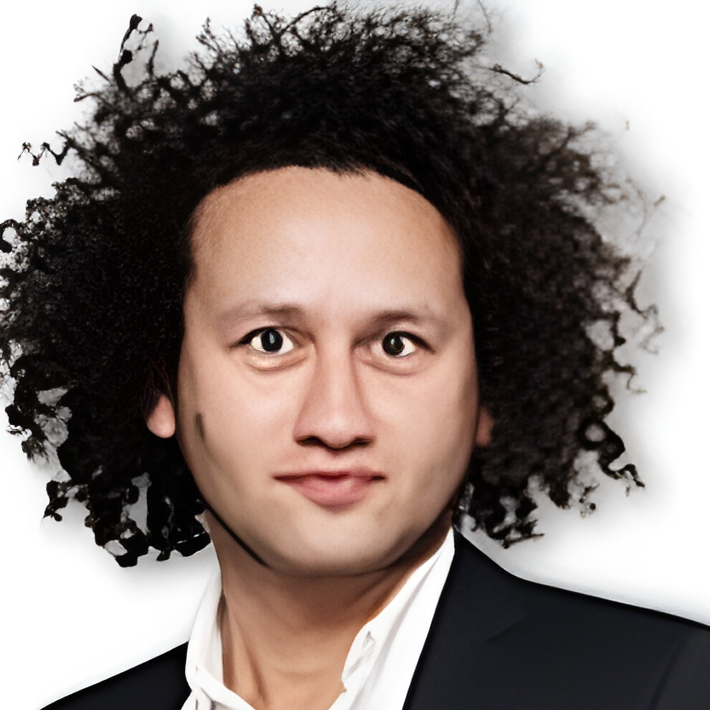
Erdal Ak
In today’s retail landscape, companies face the challenge of providing their customers with personalized and relevant product recommendations. Traditional recommendation algorithms often reach their limits, especially when it comes to niche products or context-dependent suggestions. The solution lies in the use of semantic data platforms like Almato Bardioc – a new generation of recommendation engines that focus on causality and contextuality.
Traditional Recommendation Logic: An Approach with Limits
Traditional recommendation algorithms often rely on analyzing interactions between users and products. This method heavily depends on correlations and historical data, but it often ignores context – such as time, location, or situational factors. Additionally, these systems are data-hungry, requiring large amounts of user data to make meaningful predictions. For the retail sector, which typically operates with limited data, these requirements present a significant obstacle.
Semantic Recommendations: More Context, less Data
Semantic recommendation systems take it a step further. They leverage contextual information and causal relationships to generate recommendations. Unlike traditional systems that merely recognize patterns, semantic systems »understand« the meaning of data. This enables more precise and diverse product recommendations that go beyond bestsellers, to address the so-called long tail – niche products that are rarely in demand individually, but collectively generate higher margins.
Architecture of recommendation engines based on the semantic data platform Bardioc
Three Key Advantages of Semantic Recommendation Engines:
1. Solving the Long Tail Problem
By semantically integrating contextual data, niche products can also be effectively recommended. This diversity leads to better coverage of individual customer needs and simultaneously increases revenue potential.
2. Efficient Scaling Despite Limited Data
Semantic systems do not require large datasets to provide meaningful recommendations. Contextualized information allows the creation of insightful customer profiles, even with small amounts of data.
2. Considering Temporal Aspects
Recommendations can be made time-relevant – for example, suggesting suitable products for specific seasons or age-related recommendations like diapers for babies at a particular developmental stage.
Why Semantic Systems Make a Difference
In e-commerce, marketing, or mobile applications, context plays a central role in purchasing decisions. A semantic system considers not only a customer’s preferences but also their current situation and potential needs. As a result, recommendations are not only more accurate, but also more relevant and sustainable.
The Perspective: More Context, Higher Precision
Research in semantic systems increasingly focuses on integrating new sources of context and scaling the underlying models. The goal is to make recommendation algorithms more flexible and user-friendly, offering added value to both – companies and end customers.
For retailers, the use of semantic recommendation systems provides a decisive opportunity to realize attractive business cases and stand out from the competition through innovative technology. Customers are thereby understood on a deeper level. The future lies in systems like our semantic data platform Bardioc that not only do analyze data but also interpret its meaning within the given context - for recommendations that are not just meaningful, but also extraordinary.